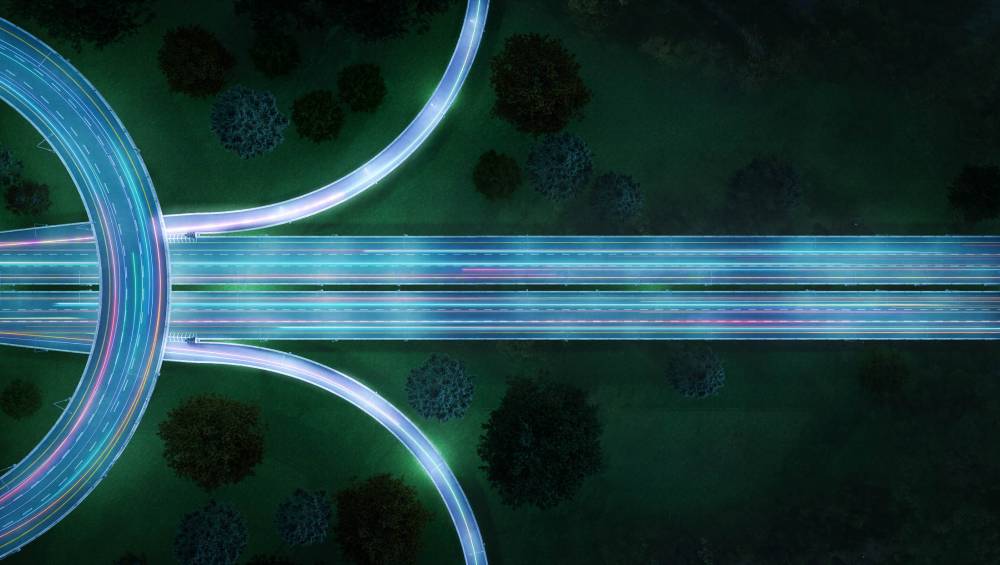
Anser® - a multi-physics optimisation engine for the new era of ML and AI
To fully leverage ML and data driven approaches, we need to step back and adapt this human-centric approach. ML does not care about graphic interfaces, human intuitive templates or ease of use. Instead, what ML primarily cares about is data, scalability and ease of integration of ML models into the computation pipeline.
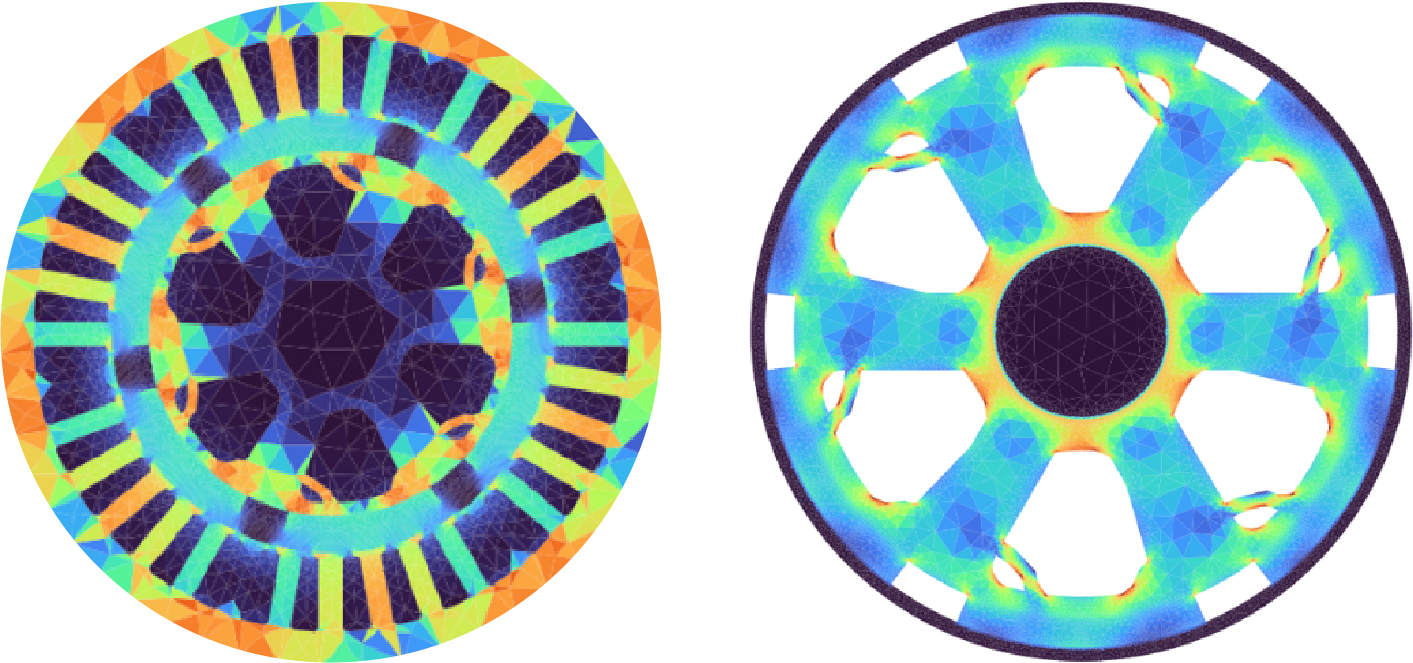
Background
In the area of engineering and applied life science research, the quest for optimised solutions is never-ending. The landscape of problem-solving has been dramatically reshaped with the advent of sophisticated computational techniques that started as simple CAD systems in the 70s and have been evolving ever since to empower engineers and scientists.
Recently, exceptional progress in machine learning (ML) algorithms have added a new powerful tool that can be harnessed to push what is possible in the design and optimisation space even further - the combination of traditional numerical methods with cutting-edge data-driven ML simulation and optimisation. This integration not only accelerates the pace of innovation but also unveils solutions that were once deemed intractable or too complex to tackle. However, traditional CAD software often struggles to leverage the full potential of ML. The main reason behind this is that traditional CAD software has been developed with human engineers in mind – the CAD software is there to assist an engineer, present the solutions and visualisation, but it is down to the engineer to make critical design decisions.
To fully leverage ML and data driven approaches, we need to step back and adapt this human-centric approach. ML does not care about graphic interfaces, human intuitive templates or ease of use. Instead, what ML primarily cares about is data, scalability and ease of integration of ML models into the computation pipeline.
Introducing Anser
At the heart of Monumo’s technology is its Anser® engine, which tightly integrates a high fidelity, multi-physics, multi-component simulator with data driven ML and cutting-edge optimisation technologies. Anser is written from scratch with data and scalability in mind, enabling the team at Monumo to generate the large volumes of diverse engineering data needed by modern ML and generative AI methods.
While Anser can address a range of complex engineering challenges, our first target is the electric motor system, and in particular, those used in electric vehicle (EV) powertrains. This application was chosen because electric motor powertrains are complex multi-physics and multi-component systems. The current tools struggle to optimise at the system level and beyond restrictive templates. The optimisation of powertrains is sufficiently complex that the application of advanced data driven ML approaches can deliver significant improvements in an aggressive timeframe. Electric motors are also crucial to society’s efforts at decarbonisation – facilitating their adoption by making them cheaper and more efficient, and ensuring that their production is less environmentally impactful is critically important to achieve our climate goals. EVs themselves represent a structural change to the traditional automotive sector with as many disruptors emerging as there are traditional players; the need for innovation is crucial.
Anser’s multi-physics simulation module enables high fidelity simulation of electromagnetic, mechanical and thermal properties of electric motor systems. We have included within the module a long list of motor powertrain specific computations, including various motor losses, control waveform parametrisation and inverter losses. We have also added a flexible, free-form parametrisation of motor geometry and control algorithm. The simulation module allows us to simulate electric motor powertrain designs in a highly dimensional and flexible design parameter space, unlocking potential to find unintuitive and optimal powertrain solutions. To fully exploit this potential, we need to explore the available design space efficiently, and this is where the ML driven optimisation module of Anser comes in.
During optimisation Anser can utilise a range of different ML techniques to speed up the optimisation process. These techniques range from neural network-based emulators for parts of the simulation computations, through dimensionality reduction to make the optimisation process more efficient, to generative models that propose candidate motor designs. Since these ML techniques make our simulation and optimisation faster, we can explore more diverse designs, which in turn generates more valuable data that can be used to improve the ML models – it is a positive feedback cycle. The more optimisations we conduct with Anser, the more data we get, the better ML models we can train and the more new designs (data) we can explore! With time, we will have accumulated enough diverse powertrain design data that we will be able to train large, generalisable models that will be able to replace costly computations and automatically generate designs to fulfil specific powertrain applications requirements like torque, volume, cost, etc.
Current developments
The challenge set was to see whether Anser could find a light and efficient motor design for use in a supercar. For this application, good designs are characterised by high efficiency and low mass, since they both directly impact the racetrack performance. A lighter motor reduces the vehicle mass directly, while making the motor more efficient (less lossy) allows for lighter cooling system and potentially less battery weight. This showcases the power of Anser to tackle complex, multi-physics optimisation challenges in high dimensional parameter spaces.
Reduction of motor mass is achieved by removing material (steel) from its rotor; however, this removal needs to be done in a way that does not compromise the motor’s structural integrity (including at high speed rotation) while ensuring there is sufficient steel left in the right places to provide the necessary flux paths that are needed for efficient torque production. To find the optimal light motor, all these considerations need to be considered and evaluated across all operating points of the drive cycle. To achieve the results shown in Fig 1. we parametrised the design with 1,080 parameters, including 2 magnet parameters, 256 parameters to describe free form rotor cutouts and 822 parameters of the drive cycle control waveforms. We simulated the motor’s electromagnetic and mechanical properties for more than 160,000 candidate designs.
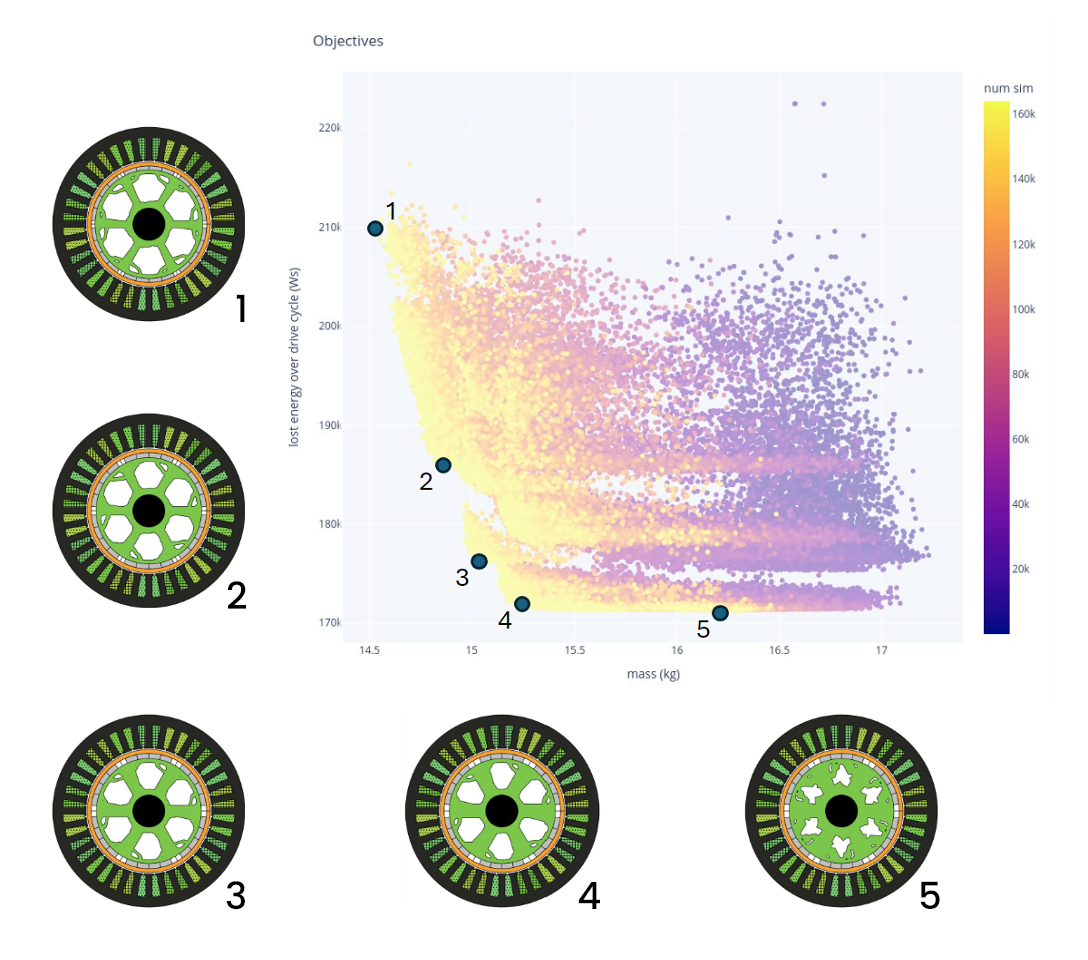
Figure 1. Optimisation results from Anser. Each point in the top-right plot is a design variation simulated over the drive cycle, taking multi-physics constraints into account. Warmer colours correspond to designs explored later in the optimisation process. A Pareto front of mass – loss trade-off is achieved and can be explored by the user. Example designs drawn from the Pareto-front are labelled 1-5.
Each point in the top-right plot of Fig 1. is a design variation simulated over the drive cycle, taking multi-physics (see Fig.2) constraints into account. Warmer colours correspond to designs explored later in the optimisation process. A Pareto front of mass vs loss trade-off is achieved, and we can see interesting designs drawn from it. Performing such an optimisation required solving over 4 million electromagnetic configurations and over 300,000 mechanical configurations in just over a day.