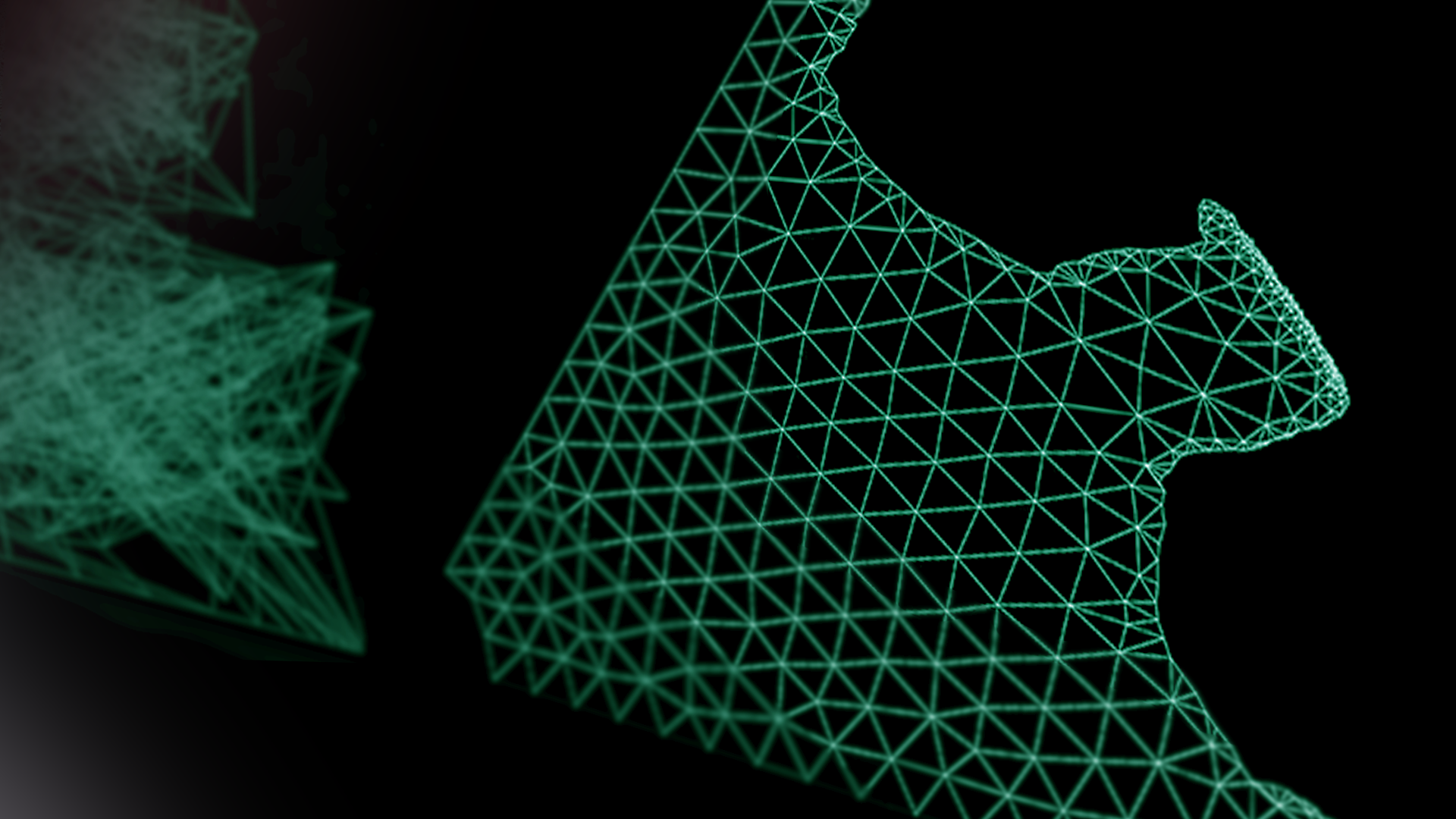
Executive Summary
With generative AI taking centre stage in many tech discussions over the last couple of years, our CTO Jaroslaw Rzepecki PhD. spoke with AI Journal about what is next for AI in a world beyond LLMs: enter LEMs.
Check out Jaroslaw’s full piece here.
Recent developments in AI systems able to generate pictures (DALL-E2) or text (ChatGPT) have taken society by surprise. There aren’t many who haven’t heard of or tried generative AI in some capacity whether that’s for the creation of personal Pixar avatars or using it for a university essay.
But under all of the fun and time-saving hacks, there have been the underlying questions of “what does this mean for the future of humankind?” and “what is creativity?”. This sci-fi story we believed to be hundreds of years away could be fast approaching.
Taking a step back, and putting the question of whether current generative AI models are creative or not to one side, let us instead focus on what potential does AI hold beyond large language models? And why did we start with text and images?
There are three main ingredients that make AI training possible and successful - access to data representing the problem domain, the ML algorithms needed to process that data, and the compute power to run those algorithms and crunch the data.
With a dramatic increase in compute power availability over the last decade, increase in cloud computing capabilities, and the introduction of transformer based Neural Networks, there is now an effective way of using large volumes of data in training. And as text and image data were readily available on the internet, this was naturally the first point of focus of the use of generative AI.
But despite the buzz around Chat-GPT-like models, we can’t forget that this isn’t the first-time modern AI has impressed by dethroning a human at something that is considered highly complex and intellectual. AlphaGo beat the Go world Champion in 2017 as researchers from DeepMind trained AI to outclass a human in a very complex game.
The key aspect of AlphaGo’s success was the ability to simulate the game, this has allowed the training process to generate the data it needed on demand. Simulating Go is very easy – the rules are simple and the winning conditions very well defined, but the success of AlphaGo suggests that if we can simulate a given process, then we can use that simulation to generate data that then can be used to train a superhuman AI to operate in that process domain.
So, what does this mean for Monumo, engineering, and optimal motor design?
At Monumo, we have developed a simulation stack that enables us to run high fidelity and a multi-physics simulation of electric motor powertrain design. We have written that simulator with scalability and data in mind which means we can run many simulations every day and each time we run it, it generates valuable data that can be used for AI training.
Let's take the example of Go: in the game we have game rules, winning conditions, and what we want our AI to find is an optimal game strategy.
Comparatively, in engineering, specifically motor design, we also have rules: the laws of physics, manufacturing constraints, and a winning condition – an optimal design for given application, and what we want our AI to do to find that design for us.
For us, we are able to use the data generated so far to train ML models that make the simulation faster, which in turn allows us to explore more design space and generate more diverse data - it becomes a positive feedback loop and the more data we have, the better we can be at generating data and pushing the boundaries of unexplored motor designs.
At Monumo, over the coming years we will have accumulated enough valuable motor data that we will be able to train models of a scale similar to Large Language Models (ChatGPT, DALL-E2-like models), we call those new models Large Engineering Models (LEMs).
LEMs will unlock for engineering and motor design what ChatGPT and Dale-2 have achieved for text and image generation. However, there is one major difference: whilst the output of LLMs mimics human output, the output of LEMs will out-perform human engineers’ output, just like Alpha-Go out-performed the human Go champion. LEMs are about to change the future of engineering and the process of invention.